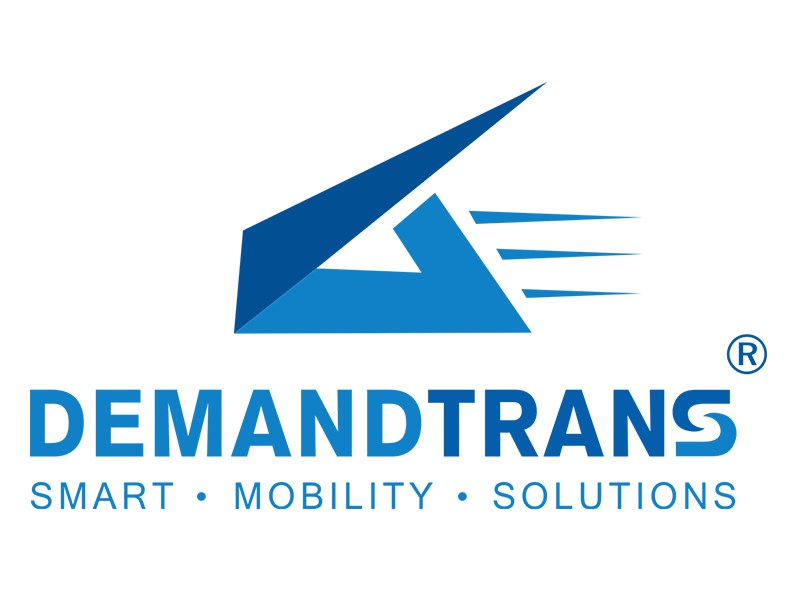
Our Transit Lab suite of tools allow us to help agencies make decisions about demand responsive transit, recently referred to as microtransit, and how these services fit into the larger regional vision for mobility and quality of life. This case study illustrates actual results from the second step of a four-step process:
1. DRT Area determination
2. Simulation of potential services
3. Validate service concept with actual dispatching tools
4. Rerun the simulation in a 4-step model at a very fine scale to understand regional mode share for more sustainable infrastructure investment
Future case studies will tackle the other three steps. With the MobilityDR software, it is possible to run on-demand services as curb-to-curb service, where customers can be picked up and dropped off at any address in the service area, or as a service where customers must walk to a nearby corner, a.k.a. corner-to-corner. Services can be designed to operate anywhere within this spectrum, as we define zones and the presence of checkpoints– or timed transfer points with other fixed route services – allowing agencies and private transportation companies to operate anywhere along a “fixed to flex” spectrum. This allows agencies to design services which are first-mile last mile, community circulation, and deviated flex-route. For any of these services, agency planners can set parameters regarding the length of deviation and number and location of checkpoints which will translate to customer level of service.
As an example, the advantage of the curb-to-curb service is that customers might find it more attractive since they can wait at their point of origin and leave when they receive a notification that the vehicle is nearby; however, this can generate longer vehicle tours since the vehicles may have to navigate one way streets or make several turns to return to main corridors in order to perform subsequent pick-ups and drop-offs. With corner pick-ups, agencies can encourage customers to congregate at intersections. These virtual bus stops could be labeled or they could be communicated to customers via the MobilityDR app (to reduce need for producing bus stop signs).
Our TransitLab tools allow us to explore all the various configurations of potential services and report performance measures important to customers and operators. Table 1 shows a comparison of mileage for 6 scenarios with varying service area sizes, checkpoint configurations, fleet sizes and anticipated demand levels.
Table 1. Scenario Performance Summary | |||||
Parameters | Performance | ||||
Scenario | Zone Requests Per Hour | Average Hourly Fleet Mileage (6 vehicles) | Average Mileage per Vehicle | 85th%ile Minutes Moving Per Hour, per vehicle | |
Morning Peak, 6 vehicles | |||||
One checkpoint, 12.2 square mile service area | 15 | 54.5 | 9.1 | 19.2 | |
30 | 100.5 | 16.7 | 39.3 | ||
50 | 142.1 | 23.7 | 57.4 | ||
Three checkpoints, 12.2 square mile service area | 15 | 58.8 | 9.8 | 21.1 | |
30 | 106.4 | 17.7 | 40.4 | ||
50 | 144.5 | 24.1 | 59.1 | ||
1 checkpoint, 14.6 square mile service area | 15 | 61.8 | 10.3 | 22.7 | |
30 | 108.9 | 18.1 | 41.9 | ||
50 | 146.5 | 24.4 | 60.4 (infeasible) | ||
Morning Peak, 5 vehicles | |||||
Zone Requests Per Hour | Average Hourly Fleet Mileage (5 vehicles) | Average Mileage Per Vehicle | 85th%ile Minutes Moving Per Hour, per vehicle | ||
One checkpoint, 12.2 square mile service area | 15 | 53.6 | 10.7 | 23 | |
30 | 95.9 | 19.2 | 46.4 | ||
50 | 131.9 | 26.4 | 64.9 (infeasible) | ||
Three checkpoints, 12.2 square mile service area | 15 | 74.9 | 15 | 24.5 | |
30 | 107.2 | 21.4 | 49.7 | ||
50 | 133.8 | 26.8 | 66.6 (infeasible) | ||
1 checkpoint, 14.6 square mile service area | 15 | 58.9 | 11.8 | 25.5 | |
30 | 104.9 | 21 | 48.4 | ||
50 | 138.1 | 27.6 | 70 (infeasible) |
As Table 1 shows, this agency can see that if they want to achieve 50 trips per hour in the morning peak for the selected service configurations, they will need to schedule a few more vehicles. MobilityDR enables agencies to achieve service productivities as high as 10 passenger trips per vehicle service hour when the zones are configured appropriately to match demand patterns. With these simulation results, we can study potential vehicle tours for dynamic services and (1) understand what the service might be like for customers and (2) get a sense of the operating costs (Figure 1) before the service is introduced. This ensures decision-makers can choose the right parameters to achieve their ridership and cost objectives at the network and service design stages. This informed decision making not only benefits the customer experience, but has the potential to improve overall network efficiency and service delivery in areas where demand fluctuates significantly throughout the day, or where travel patterns simply do not necessitate fixed route transit.
For more information, please contact us at operations@demandtrans.com
Figure 1. Regimes of Service (in minutes) versus increasing number of checkpoint stops (1-4)